In a series of blogposts I have been sharing some learnings with regards to enhancing live chat, introducing Conversational AI and scaling up with a Bot at a banking organization. Most of these learnings would apply as well for other industries where investments are made to enhance customer service channels.
In the previous post we have learned about how to facilitate your employees in order to respond to questions coming in through live chat. We have also learned what kind of metrics are important for measuring the chat performance. In this last post of the series we will dive deeper into the benefits of implementing Bots and how these fit in your contact channel strategy.
Get your bot foundation in place
Chatbots have been around for a long time but gained more traction from the mid 2010 years as chat and messaging apps have grown in popularity and more advanced bot engines where introduced. Modern bot engines offer the capability of converting conversations (text or voice) into structured data through Natural Language Processing (NLP) by taking a written or spoken input and breaking it into language objects. Natural Language Understanding (NLU) is taking care of the interpretation of the language objects by matching the intents and classifying it based on what the user wants to achieve and which sentiments the user experiences. To respond in a conversational manner Natural Language Generation (NLG) is used to turn structured data into human language.
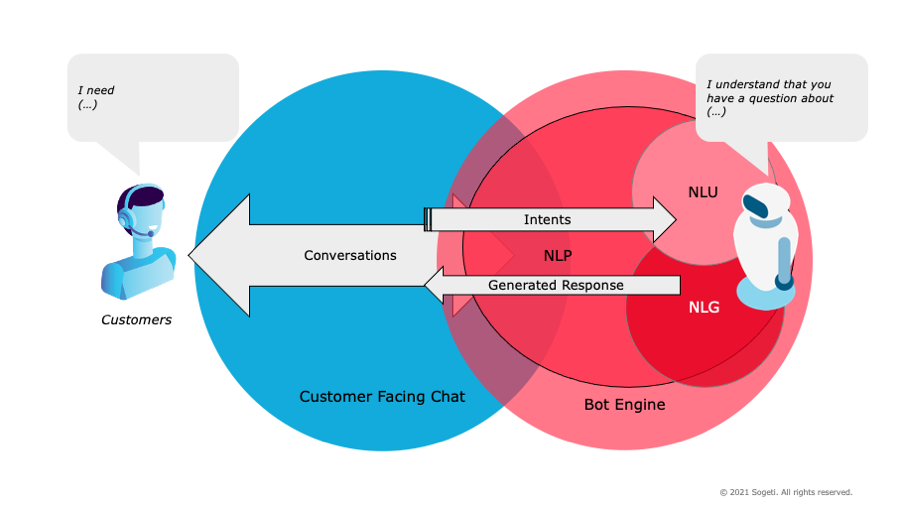
With the evolution of bot engines chatbots are getting more intelligent and no longer deliver only plain conversations for questions and resolutions but also engage, explain and mimic human language. Despite the advancements not many organizations have experienced the benefits of chatbots as implementing these could be challenging for different kinds or reasons.
Chatbot NLU engines are responsible to recognize the customer intents but how can we make this work good enough so that the bot can recognize and handle the most common questions?
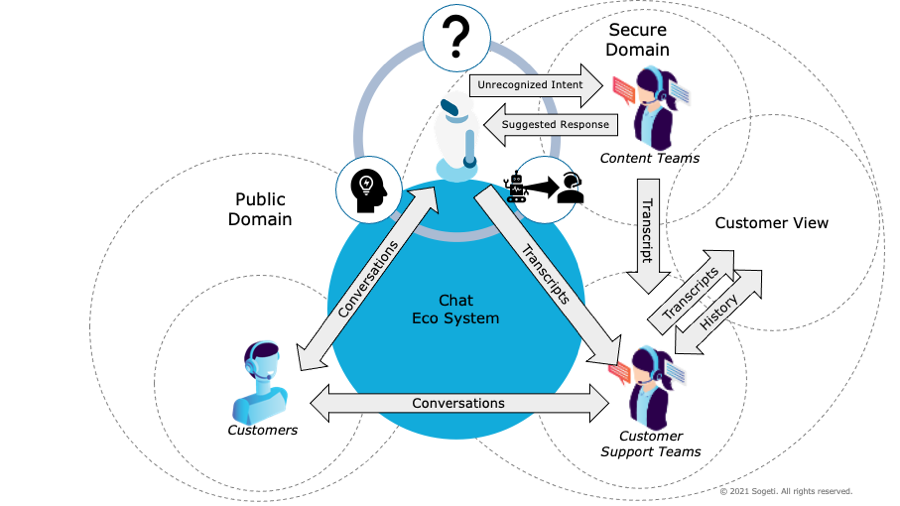
Organizations have experienced that customer intents will not be recognized properly without content moderation, orchestration and training efforts. Even though machine learning helps to learn from patterns and previous experiences a huge amount of training/conversation data and skilled (human) specialist are needed to improve the understanding skills of the bot. Bot engines heavily rely on content components and the way these are implemented can be proprietary to the platform that you are using. Examples of content components are taxonomies, conversation flows and tagging mechanisms. In a lot of cases manually orchestrating the dialogues and matching unrecognized intents is still necessary. Setting up the content moderation and orchestration is part of your content live cycle management and requires involvement of taxonomists, conversational designers, copy writers and also customer support professionals.
They need to analyze the customer intents, constantly measure the overall chat performance and then, in consultation with the DevOps– and Product Management teams, gradually scale up the number of interactions with the bot.
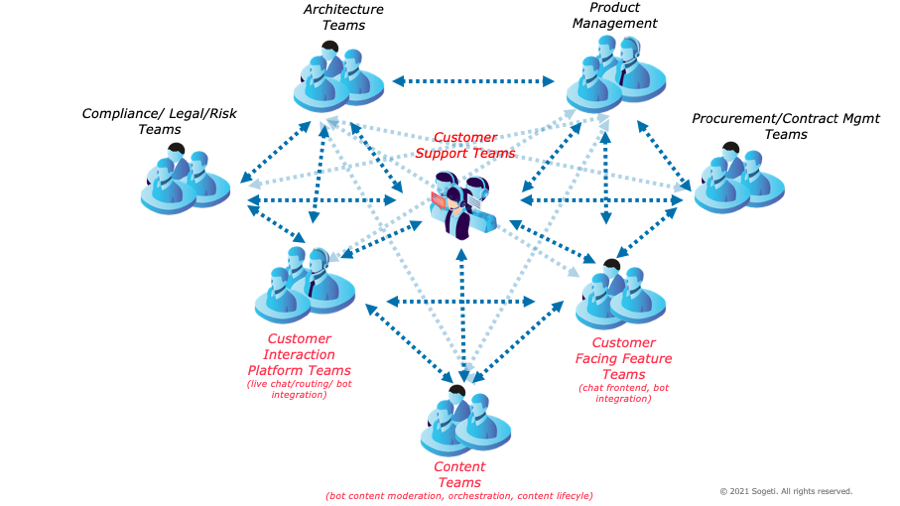
In this way adding chatbot intelligence can be instrumental for keeping support mechanisms on par with expected service levels. The better these professionals are playing together the better the potential of your bot can be utilized. As the bot handles more conversations, reporting and training capacity might be increased by involving data engineers and dedicated bot coaches. Work together with your customer support organization to experiment and scale up. This way your chat bot is becoming like a virtual member of your team which will get better if you give it the right attention.
On the technical side DevOps feature teams need to be able to easily integrate chat features with bot engine API’s and Live Chat systems. To experiment with bots in the different customer journeys a flexible architecture is required to plug in different Bot Engines. Conversational platforms and Bot engines in specific, have started to evolve into more loosely coupled systems. Vendors are offering SaaS platforms with APIs and connectors allowing flexible integration but be aware of a vendor lock-in. Your solution architects need to consider the technical integration parts but also look at the content related components (discussed earlier) to support content lifecycle management activities. An important question to keep in mind is what the migration path would be towards implementing alternative platforms as part of your exit strategy.
Over time the nature of your Bot(s) could be changing from handling FAQs to supporting (sales) transactions, giving advice or offering specific services. Select your platform vendors and integration partners wisely. Make sure that they can innovate and bring value by innovating and scaling up together with your organization. This means that as you are processing higher volumes different support tiers are applicable and 24×7 availability should be covered. Involve your contract management teams to measure the actual load so that they can validate the reported volumes on the vendor side. But also stay in touch closely with your vendor to understand their platform roadmap.
As you are planning for more personalization options in your bot(s), make sure that vendors can keep up with the compliancy and user privacy demands. In this case involve your CLR teams before every scale up step. With regulations such as the GDPR, companies must be cautious when they collect data on the Vendor side. Examples of compliancy demands are that conversation data needs to be masked to prevent any disclosure of Personal Identifiable Information but also defining the retention period for storing historical conversation data.
The baseline should be that your conversational AI initiatives can be defined as experiments with clear goals and successfully scaled up as soon as the value has been proven. By getting your bot foundation in place, scaling up (and down) can be done in a controlled manner. Do not think of implementing bots as a cost saving initiative for the short term as you need to invest in new technology but also specific knowledge next to your existing initiatives. However, there are some clear advantages on a short term, and I will touch upon this in the coming paragraphs.
Improving on the responsiveness
A bot can instantly respond to any questions coming in from the customer. If well trained, a bot can handle most of the repetitive questions. The goal should not be minimizing human interaction by implementing bots but making sure that a customer gets answered quickly and adequately.
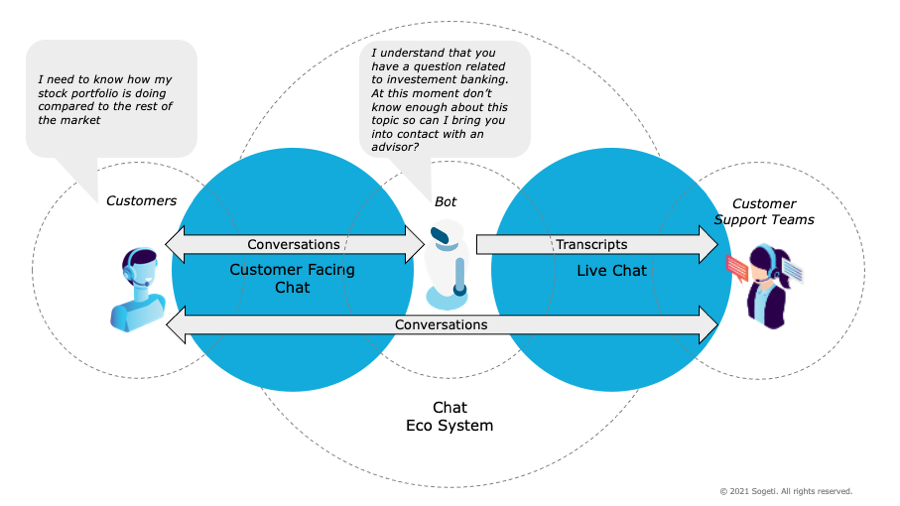
For the unrecognized intents you still need to offer an escalation option to an employee. As customer intents can be recognized by the NLU engine, context variables can be derived from the conversation and help with routing a conversation to the right employee in case of an escalation. Setting up the routing mechanism and intent mapping requires involvement of your live chat platform teams, conversational designers, taxonomists and solution architects.
Are customer support team employees still capable of helping the customer by understanding the context of the question and having access to customer details at any time? A conversation that is being escalated should provide enough context for the employee to understand the question so make sure that transcripts of conversations are handed over as well.
Improving on the findabilty of information
As conversation topics may vary, the customer intents coming from the bot can in this case be helpful with pointing out the customer to the right information, self service page or even integrate with AI Search. Eventually your bot could replace the more traditional interfaces for FAQ-and Search functionalities but only do so if it has been proven that your bot can offer a better customer experience.
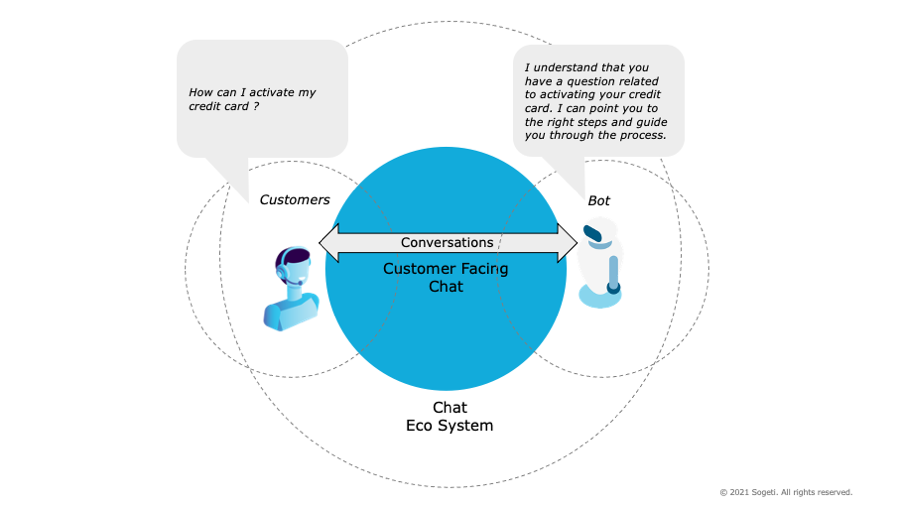
Reducing customer service calls
To find out whether a bot has impact on the call reduction numbers you need to experiment with this in your live chat contact channel(s). And with experimenting I mean that you are actively monitoring the performance of your chatbot as a first point of contact. You can test this scenario on a certain percentage of your chatting customer and scale up gradually if the customer experience is acceptable.
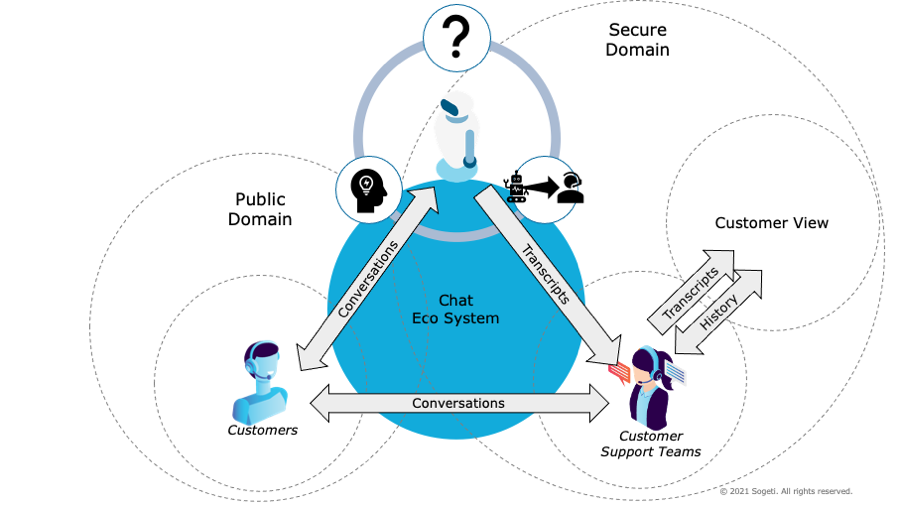
Important metrics to watch on the total volume of processed conversations are the escalation rates (total number of handovers from bot to employee) and the percentage of incomprehensions (the percentage of unrecognized intents). Also watch these metrics in relation to the first contact resolution (total number of issues resolved on the first call) and other operational call center metrics.
Trend data related to the number of handovers to employees is essential to validate if the bot can handle conversations without any escalations. A higher number of incomprehensions could mean that the bot needs to be trained better on popular intents to eventually reduce the escalation rates. If the number of escalations is reduced it frees up time for the customer support teams to handle the more complex questions. Be aware that this will impact the average handling times of the conversations by the employees, but this would not be an issue if the total number of calls will also be reduced. If the average handling time does go up this could be a good reason to rethink on how to better facilitate the customer support teams. In the previous blog post we have covered some basic things that need to be in place. The next generation customer interaction platforms offer more extensive support for integrating with conversational platform API’s, knowledge management systems, applying Robotic Processing Automation (RPA) and even measuring the employee experience. An example of applying RPA could be to reduce the handle time of key enrolment processes.
As your customer support teams are the first in line to communicate with the customer they have lots of knowledge about the experience so listen to them, keep them involved but also facilitate them with customer experience data. Involving them means that you should define pilots and experiments together to better plan and prioritize the roadmap for your live chat and bot enhancements.
This is my last contribution to Sogetilabs and I would like to thank the team for offering me a platform to share my ideas and experiences over the last 7 years. Also thanks to Emil Wesselink and Chris den Arend for sharing their feedback and suggestions. For whomever wants to keep on following me or stay in touch feel free to connect with me on Linkedin https://www.linkedin.com/in/thomaswesseling/
Sources
https://www.cognigy.com/news/10-ways-to-improve-first-call-resolution-with-conversational-ai
https://medium.com/mysuperai/what-is-intent-recognition-and-how-can-i-use-it-9ceb35055c4f
https://www.forrester.com/report/Stop+Trying+To+Replace+Your+Agents+With+Chatbots/-/E-RES143033
https://www.forrester.com/report/Build+An+OutsideIn+Contact+Center+Roadmap/-/E-RES84261#
https://medium.com/sciforce/a-comprehensive-guide-to-natural-language-generation-dd63a4b6e548