In a series of blogposts I will be sharing some learnings with regards to enhancing live chat, introducing Conversational AI and scaling up with a Bot at a banking organization. Most of these learnings would apply as well for other industries where investments are made to enhance customer service channels.
Before enhancing live chat, you need to know where in the customer journey chat is offered as a contact channel. On your public website or in a secure environment where the customers are signed in? In the previous post I have elaborated on live chat and how this can evolve as part of your contact channel strategy. In this blog post I will dive deeper into measuring the chat performance in order to anticipate on customer/employee input and events. How responsive is your organization towards customers? Are all chat conversations handled by live agents or also by automated systems (like i.e. bots)? Are you capable of measuring the volumes and capturing operational performance data? Let’s start with getting a better understanding of how your employees are facilitated and then dive into measuring the performance.
Facilitating your employees
As part of your workforce engagement management strategy, creating a supportive workplace can have a direct impact on the quality of customer service. This includes a proper onboarding and basic training for call center employees with insights on the customer psychology and the customer environment. Employees should know where information can be found, how (self) service solutions can be used from a customer point of view and how customers with different backgrounds think and behave.
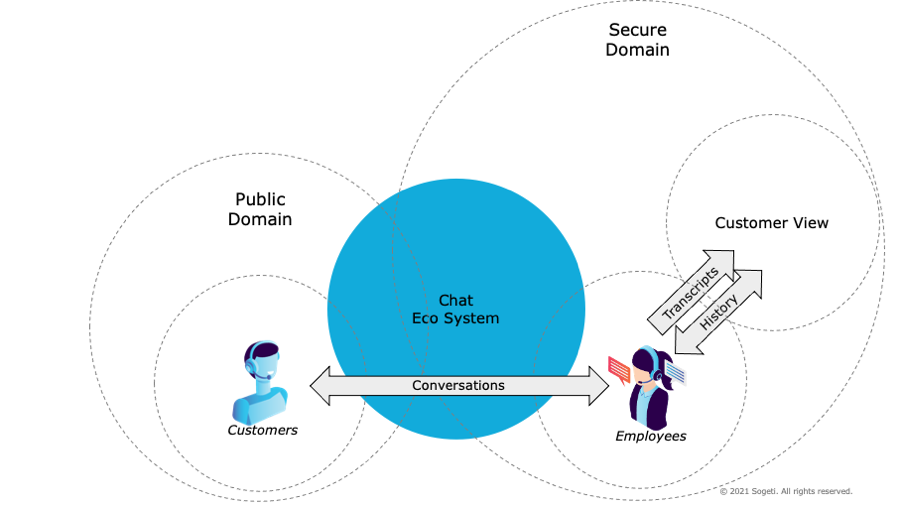
Assuming that live chat operates in a secure context and that the customer can be identified, employees need access to a single customer view (dashboard). As the customer view requirements could be different per organisation I will focus on a few necessary requirements. From a support perspective a customer view dashboard should help with keeping track of earlier customer interactions, finding out what the context of the question is and (if applicable) the status of any unresolved cases.
With a layered support model in place, reaching out to other employees or a second line support needs to be possible. Depending on the amount of products and services offered and the complexity, specific knowledge might be required to resolve the more complicated questions. As mentioned earlier live chat backends should be capable to support routing and queueing mechanisms to make sure that conversations, initiated by customers, are routed correctly. And if the routing works correctly, handing over questions should be minimised.
Measuring the live chat performance
Measuring the operational performance of the call center can be done through a wide set of metrics. The summary below is not exhaustive but should focus on the important ones.
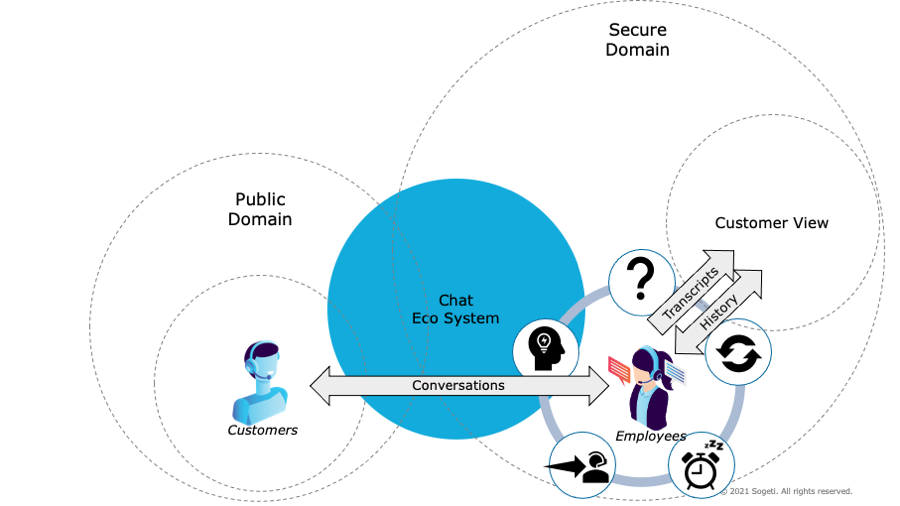
Operational call center metrics to watch are the call or conversation volume (total number of customers initiating live chats), abandon rate (percentage of customers who abandon the queue before being served), first call resolution (total number of issues resolved on the first call) but also average handle times (average time to handle a call from start to finish).
An increase in conversation volume and abandon rates translates into a declining service level. Real time monitoring of the volumes can help with detecting any possible disruptions elsewhere (like i.e. unexpected down time of a specific service).
Under normal conditions, if issues are difficult to resolve you would see some metrics going up such as the number of repeat calls (total number of issues unresolved), average waiting times (average time that a customer has spent waiting) and tranfer rates (the percentage of transfers on the total volume). This could very well indicate that self-service (content) needs to be improved. In case no self service process is available, additional training could be needed or routing can be improved.
Implementing in-app analytics
Measuring the technical performance of your chat front-end can be done by implementing in-app analytics. In this case metrics are collected in analytics tools such as i.e the Adobe, Google Analytics or other related solutions. Most of the analytics tool require embedding of an SDK component in your chat features which can be available cross platform. With this your are able to receive out of the box analytics data but on top of that you can manually add events in your app that generate specific in-app metrics. This allows you to get both cross platform metrics all well as specific in-app metrics per platform type. Examples are real-time traffic, button presses and funnel metrics for conversion data reports. Before implementing in-app analytics make sure that you discuss with your legal department on the data that you can or cannot collect and how to inform the user about data collection in your app. Due to privacy rules, storing personal identifiable information is not allowed without a user consent.
Measuring the customer experience
In order to measure and collect experience metrics, you need to setup a feedback loop. This can be done for both the customer and the employees handling the chat.
Let’s focus on the customer side first and how to setup this feedback loop for live chat. Measuring the customer experience can be done through collecting mood ratings and open feedback at the end of the chat session. To make this experience less intrusive for the customer, feedback options should be optional and not require more than 2 or 3 steps to finish. Collecting feedback from the employee side can be done by injecting a feedback component in the live chat console. The input can be more or less the same.
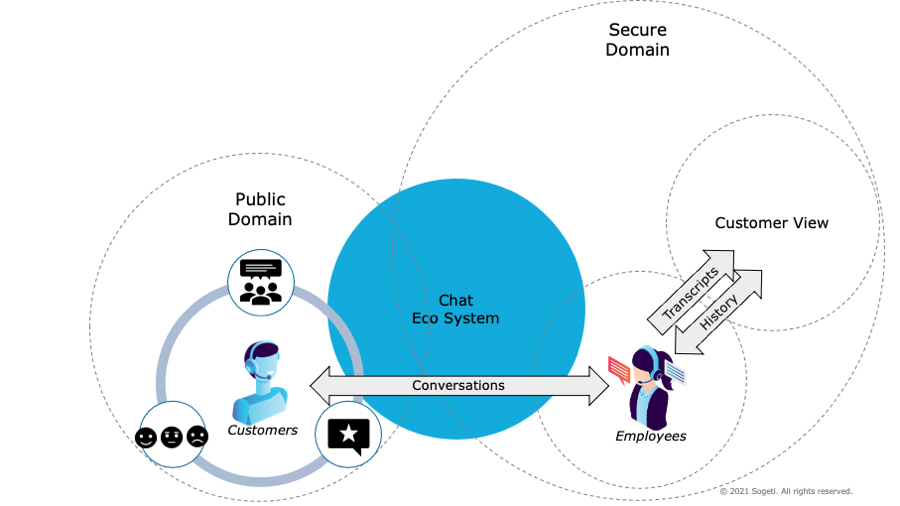
Collecting mood ratings will be more useful if you are able to drill down into any related feedback. To deal with a growing amount of diverse feedback data, consider utilising text mining tooling for analyses. This is useful to detect trends and (in the long run) will allow you to categorize the feedback automatically. Examples of categories are buckets for technical issues, most popular customer intents, the way questions are handled, user interface improvements or even feature requests. Alternatively online surveys can be offered randomly at the end of a conversation or a transaction.
The purpose of setting up this feedback loop is that you are able to find out what the top feedback items are and define actions for improvements. In the end this is helpful with defining the roadmap and prioritiziation of your backlog.
Dealing with the unpredictable
Customer support organizations have been adapting constantly to serve the the customers who are increasingly diverting to online channels. At the same time employees have diverted to working remotely. All this has resulted in a heavier utilization of online contact channels while being under pressure to scale up (cost) efficiently.
As the conversation volumes are volatile due to seasonal influences and disrupting events (such as a pandemic), forecasting could be challenging. Despite the effort of monitoring and analysing, unpredictable events will occur. In order to adapt flexibility is desired. How can we deal with this unpredictability and keep the responsiveness on par? Besides scaling up the call centers with more people what other options are available? More about this can be read in Part 3 where we will focus on adding conversational AI.
Thanks to Emil Wesselink and Chris den Arend for sharing their feedback and suggestions.
Sources
https://www.callcentrehelper.com/best-practices-to-improve-live-chat-120603.htm
https://www.nextiva.com/blog/call-center-metrics.html
https://www.callcentrehelper.com/metrics-best-monitoring-chat-132603.htm
https://www.callcentrehelper.com/workforce-engagement-management-131163.htm
https://www.mckinsey.com/business-functions/operations/our-insights/boosting-contact-center-performance-through-employee-engagement
Thanks for sharing such an informative blog.