Primary author: Lorrie Straka

Golden Rules and Data (Lakes)
In today’s tech industry there are many buzzwords — you may have heard things like Big Data, data lakes, machine learning, and of course A.I. You’ve probably even considered implementing one or more of these things in your own business processes. And you should! But how? The crucial first step to any successful data science project is (you guessed it) data. Data is the essence of data science — after all, it’s right there in the name! In a world of ever-increasing volumes of information, it’s vital that we find fast and efficient ways of storing, processing, and analyzing data before we can even begin to model, predict, and learn.
On Data
It is well worth the effort to consider the type of data you will need to accomplish your goals. Think about the data you currently collect and store and what additional data might be available to you. After all, your predictions and results are completely dependent on the type, quality, and amount of data you have at your disposal. For any machine learning problem the age-old axiom is: “garbage in, garbage out”. Start collecting your data well in advance of your planned data science project start date or you may find yourself taking a trip to the dump.
Consulting a data engineer on data storage and management can be useful in your planning stages. You can focus on the recommended approaches tailored to your situation. If you are short on data, it’s not always a deal breaker because approaches are available that can alleviate this shortage including transfer learning, augmented data, and synthetic data. These are all topics that deserve their own blog articles and other colleagues will talk more in-depth on these subjects.
A big question is how to store and organize data before and after you start using it for any task. It is common to use a database, which is highly structured (the formats are all known and tidy) and welcomes predictability from your queries and processes but gets pricey and sluggish if you require a lot of data retention. For small datasets, a database can be sufficient and reliable but if you’re interested in growing your data, already have a large volume of data, or plan experimental use cases such as predictive analytics, you should definitely consider using a cloud platform.
The Data Lake
Enter the data lake. Quite simply, a data lake is a storage repository for all of your data: yes, all of it. Data lakes allow you to store every type of data in one space without conflict, including raw, unstructured data, communications, log files, spreadsheets or CSV files, and even images and audio (just to name a few). The do-it-all storage.
A data lake can offer your business some additional benefits:
1. Consolidation. As mentioned above, all of your data is stored in one place. Mix and match your data from different sources when you want to perform analysis or run a model. You won’t have a plethora of different databases and storage systems, each with their own security policy, strewn across your company anymore. There will be one security policy.
2. Scalability. Data lakes are highly scalable and good thing: being able to store such raw data takes up more space than simply storing structured, processed data. These days, cloud storage is quite cheap and your data can be retained indefinitely.
3. Accessibility. Storing your data in the cloud offers the additional benefit of always being remotely accessible. Gone are the days of emailing Excel spreadsheets back and forth. Everyone will get their data from the data lake, and everyone will know where to find the data they need which makes collaboration even easier. You’ll also have happy data scientists!
4. Reusability. Because of the raw nature of the data, you can store it before you have finalized how you will use it. This means you can use the same data sources for a multitude of purposes and scenarios. Cleaning and analysis are done on request, when you query the data. Incidentally, this also makes raw data ideal for machine learning. And did I mention you have all of the data, even though you might not have envisioned how it would be used?
Though raw data itself is not immediately usable for analysis, a data engineer or data scientist can access the data and prepare it for your business analysts. The best long-term solution is to develop an automated ETL pipeline that connects to your data lake, extracts the relevant data, processes it, and provides fit-for-purpose datasets at the push of a button. If you have existing processes that already have well-defined data, it’s even easier to move everything to an automated platform that uses a data lake as its source. Fast, efficient, consistent, reproducible, and modern!
Data lakes are used with great success in many industries, including transportation, medical, education, and finance. Though you could use any storage platform to make a data lake, the big cloud platform providers also offer products that streamline the process (AWS Lake Formation and S3, Azure Data Lake are two of the biggest).
The Round Up
Whether you are just getting an idea for a project or looking for a full-scale production implementation, there are many things data engineers and data scientists can offer you. It’s always wise to consult a data scientist about what is currently possible with your data. A non-exhaustive list of options they can help you with includes proofs of concept or hosting a hackathon, both of which will generate great ideas and start your adventure in data science off with a bang. Or, naturally, engineers and scientists can be there every step of the way bringing your product to production. But the golden rule is: data first!
Author Profile
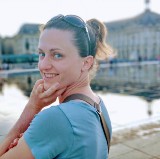
Lorrie Straka
Senior Data Scientist at Sogeti with a background in astrophysics.