Co-authors: Shreyans P. Bathiyan, and Umesh Vikram Singh
- Introduction
Advanced Analytics is rightly serving for Predictive maintenance of the system or machines and has a wide range of industrial applications in operations planning for enhanced business benefits and overall throughput. Existing static predictive maintenance systems are typically in a form of point/atomic solutions. It lacks in the effective use of maintenance history and operations knowledgebase hindering the ability of increased throughput by dis-allowing the existing system to learn from its previous knowledgebase. Recent advancements in big data technologies allow us to ideate, build and design predictive maintenance system where equipment can be scheduled to condition-based maintenance. Therefore, such cyclic, fully automated, adaptive, truly distributed, next-generation predictive maintenance systems (Automatic Process Monitoring and Predictive Maintenance) are gaining increased attention in most manufacturing environments as a means of reducing equipment maintenance related costs and downtime.
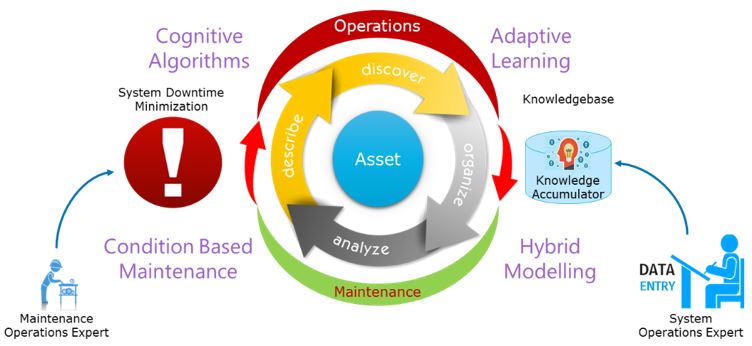
Figure 1: Next Generation Predictive Maintenance
The proposed system depicted in Figure 1 exploits uses of existing predictive maintenance system along with new information as it becomes available from previous maintenance cycle to redefine/re-estimate the equipment’s remaining useful life (RUL) and associated cost and risk factors for the next cycle. It also accounts for monitoring and controlling the maintenance cycle along with operations control based on the asset health predictions.
2. Present Scenario
Current application of predictive maintenance analytics in industry is being used to ensure minimal loss of time and resources and can be depicted as ‘routine maintenance systems’ illustrated in Figure 2.

Figure 2: Existing Predictive Maintenance System
These maintenance systems which includes typical information/data flow from asset to data lake/ cloud servers to the predictive analytics engine. Advanced ML/AI algorithms and hybrid models help to estimate the health of the asset and enable to predict the next probable maintenance cycle. These analytics engines are typically complex functional blocks having combinations of hybrid and heterogeneous models, cognitive algorithms, and rule-based engines. Upon receiving the notifications from analytics engine based on forecasted RUL, appropriate alerts/notifications details will be shared manually with the operations and maintenance team to take further action. However, all the knowledge base generated out of these actions and operations is not integrated in the next cycle of asset assessment through an analytics engine.
3. Our Proposition
In our attempt to design the next generation solution, the present predictive maintenance system is integrated with various other components to ensure automatic and uninterrupted predictive analytics for equipment’s condition-based maintenance. These include operations control processes, maintenance control processes, corresponding database and knowledgebase generated thereby, adaptive learning mechanisms, etc. And this provides the ‘adaptive’ predictive maintenance feature to the system.
Maintenance and operations logs are further used as an integrated knowledge base for analysis to improve the predictive model performance. This will ensure that at the end of each maintenance cycle, the proposed system will improve the predictive model performance based on the inputs from previous input data from maintenance and operations knowledgebase. Model performance evaluator use these logs for evaluating the existing predictive model performance by comparing the model result with the actual results. This is depicted in Figure 3.
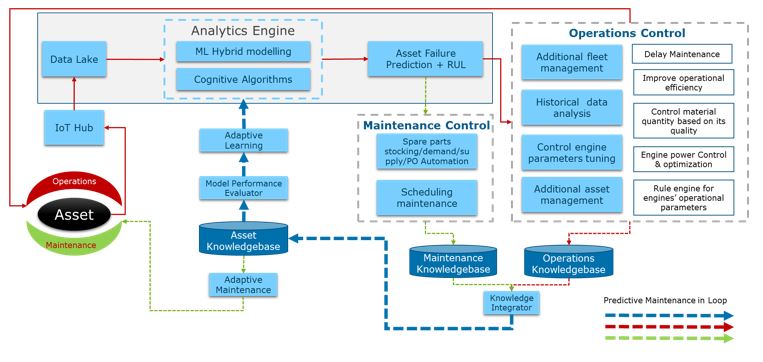
Figure 3: Asset health analytics with adaptive operations and maintenance controls
3. 1. Adaptive operations control
Notifications from predictive maintenance system will be used by the operations team to make alternate arrangement of assets to ensure the uninterrupted continuation of existing operations. This also includes additional alternate fleet management and optimization of operator utilization.
Additionally, control engine parameters can be tuned to improve the asset performance based on the alerts from the existing system. Parameters tuning will help us in delaying the maintenance thereby improving the operational efficiency of the asset and minimizing the frequency of occurrence of the maintenance cycle. Such condition-based maintenance strategy monitors and controls the actual asset health and decides which control parameters needs to be tuned.
RUL and wear & tear alerts from existing system can be very useful to maximize the operational life of the asset by balancing the payload quantity based on payload material properties. The operations team can use notifications/alerts efficiently to optimize the engine power control thereby improving the engine life and delaying the scheduled maintenance.
3.2 Adaptive maintenance control
Notifications & alerts from the existing predictive maintenance system about the RUL and asset failure are also used by the inventory management system to stock the required spares in advance. It will further ensure automatic alert generation for purchase orders of the required inventory from the suppliers. Also, other low priority pending activities corresponding to that asset can be scheduled in parallel during this non-availability of the same. This will help us in prioritizing the equipment maintenance schedule thereby minimizing the overall equipment downtime.
All such operational tuning details combined with maintenance knowledgebase can be archived as a single asset specific knowledgebase. This historical knowledge will then use for performance analysis and comparison of similar assets. This will help us in improving the predictive power of the model thereby increasing the overall operational throughput.
4. Potential Business Impacts
Predictive maintenance of an asset/equipment closely integrated with operations and maintenance cycle will soon become an integral part of manufacturing. This will greatly influence competitive priorities and hence the business strategy directly in a positive way. A proposed novel approach for enhanced, adaptive, and dynamic management of asset’s performance is up for a further exploration of its industrialization. The main challenge in offering predictive maintenance in real-time is in having a workforce large and flexible enough to meet this demand. This approach of a closed loop, automatic, and intelligently evolving solution in real time for condition-based maintenance has a wide scope of usability in industries ranging from automotive, mining, healthcare, farming domains, for example. This also can be extended to assembly lines with the end goal of improving the operational efficiency of the assets and minimize system downtime ultimately intensifying the throughput.
Authors Profile
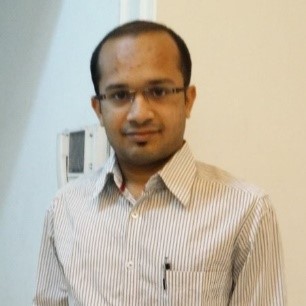
Shreyans P. Bathiyan Consultant, Digital Engineering and Manufacturing Services, Capgemini, India Consultant with over 7.5 years into solution software development lifecycle centered around data science, data analytics, machine learning, machine vision, deep learning, edge analytics offerings across spot market, mining, healthcare, energy and utilities market domains using open source tools such as R, Python, and Azure Cloud.
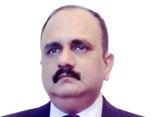
Umesh Vikram Singh: Vice President, Digital Engineering and Manufacturing Services, Capgemini, India Over 32 years of multi-sector industrial experience delivering innovative solutioning and industrialization of cutting edge technologies spanning IoT, M2M, engineering analytics, process and machine automations, sensing and controls, Product and system engineering. Responsible for the growth and support of Engineering Analytics Practice. Managed Innovation Centre and Analytics Centre of Excellence.
Pls advice Predictive maintenance record to be preparation in the foundry division compressor