NO MORE WHINING
November 20, 2019
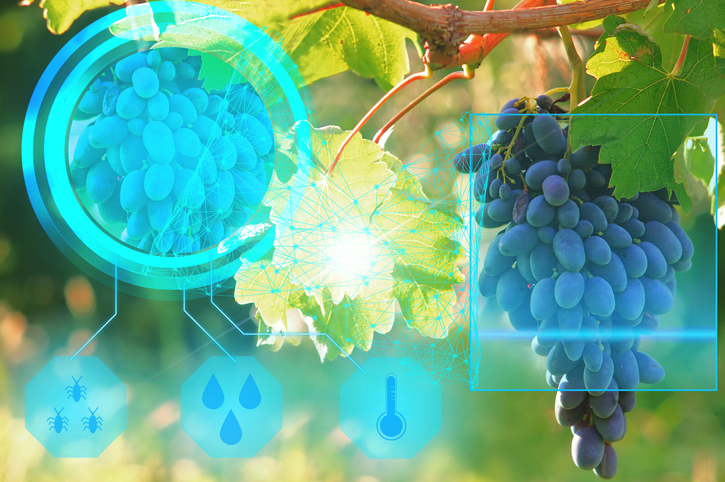
How AI and advanced Computer Vision are revolutionizing the wine industry.
French wines are admired globally for their exquisiteness. Wine is integral to the French culture and the country has a long history of wine production. France accounts for about 16% of global wine output. The export sales of French wine reached 12.91 billion euros ($15.94 billion) in 2017 (Reuters).
Capgemini – Sogeti has been a pioneer in R&D based solutions for the agricultural sector. Demonstrating its forte and research interest in the IoT CBioTS platform in recognition of complex images in vineyards, Capgemini – Sogeti in collaboration with the Château-Talbot vineyard in Bordeaux, has been carrying out an experimental project for disease detection and yield calculation.
For a vineyard like Château-Talbot, the first step in the evaluation of the wine value chain involves the calculation of the yield per plot and individual variety of grapes. This assessment is even more important considering the life cycle of wine through blends and assemblies obtained from productions spread over several years. Currently, the performance is being evaluated by two methods, a) the qualitative part, which involves chemical analysis of samples of the annual production and b) a quantitative evaluation of the annual production per plot by the technical expert of the vineyard, with a 10% to 15% margin of error. Capgemini – Sogeti proposed a unique solution to this problem of yield calculation in a more precise and reliable way, with an accuracy rate of more than 90%.
The experiment involved calculating the number of grapes per cluster and per plot using cutting-edge technology of object recognition in photos taken under natural conditions without specialized shooting devices. Moreover, the solution provides an embedded use of robots traversing the plots. According to experts, an estimation of the yield done from grain count is far less reliable than if it is carried out from an exhaustive counting from each of the bunches per plot. And finally, Capgemini – Sogeti aims at making the solution meet the second requirement as well, which is the detection and prediction of certain diseases along with the detection of the insects that act as vectors. The solution to this issue was achieved by the analysis of the appearances correlated with environmental data reported at the level of the CBIoTS platform by different sensors arranged on the plots.
For the period between Sept. 10 and Sept. 20, 2018, about 2000 photographs of three different varieties of grapes viz. Cabernet Sauvignon, Merlot and Petit-Verdot were taken with standard cameras without any specialized device for improving stability or light. These conditions were decided on purpose, to validate the performance of techniques and recognition technologies available on the shelf and/or that we would be able to develop ourselves. Given the total masking of the grain, the interior of the bunch or the total covering by elements of the environment (leaves, branches, etc.), our algorithms must recognize more than 98% of the grapes detectable on the photo of a bunch, to reach our global goal of 90% reliability for yield calculation.
Over the last decade, umpteen image processing and deep learning methods have been developed for tasks related to counting objects in general and fruits or crop in particular. However, the accuracy level of the counts is strongly influenced by the shooting conditions. The stability of cameras, lighting conditions, overlays, shadows, reflections and other noises, for example, pebbles that can be confused with grains, are all factors that greatly diminish the effectiveness of the most powerful algorithms like Mask R-CNN and its derivatives. Indeed, the application of these algorithms on our photos does not exceed 60% of the grain recognition rate.
To achieve the goal, Capgemini – Sogeti developed a revolutionary home-based recognition process combining the latest advancement in “deep learning” and innovative strategies for image processing chains, before the application of models (neural networks) in parallel, and for the fusion chains of outputs of these models. Thanks to this ground breaking work, our recognition rates are currently a little over 95%, for a learning base of less than 100 photos. We will easily reach 96% – 97% accuracy by simply increasing our learning base. By improving the parallelism and sequencing of model output mergers, we are all poised to reach the goal of achieving 98% accuracy.
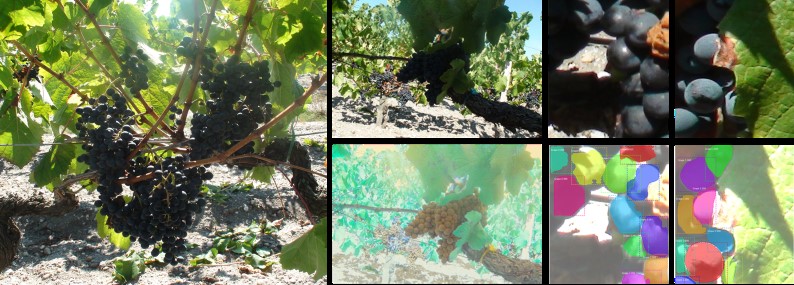
This breakthrough technology can be easily generalized to other fruit and crops. And more widely, with minor modifications, this method can be transposed to the counting of any type of object from photos taken in natural conditions without any specialized device. This now allows us to detect and count the abnormalities in leaves or fruit, symptoms of diseases, and the detection of certain insects that are vectors of such diseases.
Capgemini-Sogeti’s innovative solutions have revolutionized and transformed agricultural, industrial, engineering and bio-medical services and operations. Responding to the global challenges in the rapidly evolving food, industry and medical sectors, governments are pursuing modernization projects to optimize performance in these sectors. As a partner in these initiatives, Capgemini-Sogeti innovates, supports and guides programs through their entire lifecycle, and creates meaningful results for the ultimate end-users. This breakthrough innovation using advanced AI and Computer vision technology has a promising potential to transform not only the agricultural sector but other domains as well.