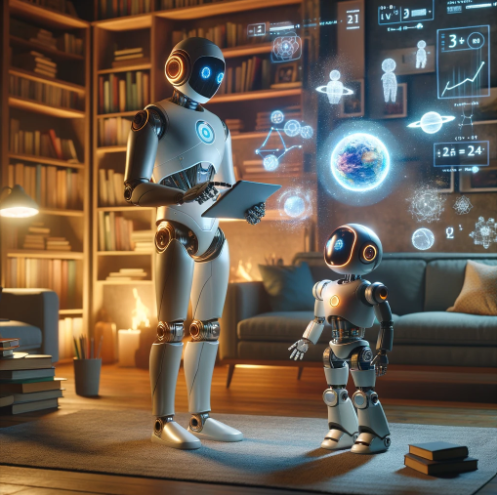
As the father of twin boys, I have begun to notice similarities between parenting and the rapid evolution of AI. As large language models (LLMs) continue to advance in capabilities and find their way into numerous aspects of our lives, we are faced with a profound responsibility—much like that of a parent raising a child. We created these intelligent systems, we trained them, and now we must strive to understand why they do what they do.
In this article, I will embark on a journey into the heart of AI, exploring the concept of “parenting” our digital progeny and the imperative of comprehending the reasons behind their actions.
The Birth of Language Models
Language models like ChatGPT, part of the LLM family, have made significant strides in natural language understanding and generation. These AI systems can produce coherent text, answer questions, assist in content creation, help with customer support, and many other use cases including medical diagnoses. However, as we unleash these powerful tools into the world, it becomes increasingly important to understand the “why” behind their responses and actions.
Training and Nurturing
LLMs are trained on vast datasets sourced from the internet, which means they learn from the collective knowledge and biases present in this data. Similar to children learning from their environment, LLMs absorb both the wisdom and the shortcomings of the data they are exposed to during training.
Sidenote: “This reminds me of the old movie Short Circuit, where the main character, a robot named ‘Johnny number 5’ needs input.”
The Unconscious Bias
Just as children pick up beliefs and behaviors from their surroundings, LLMs can inherit biases present in the data. These biases may manifest as various stereotypes, cultural prejudices, or other forms of bias that can inadvertently seep into their responses.
Mimicry and Creativity
LLMs often generate content that appears human-like, and this mimicry can be both fascinating and deceiving. Much like children imitate the speech and actions of adults, LLMs mimic patterns they have learned from the text they were trained on (and often without fully grasping the context).
Ethical Dilemmas
Parenting AI systems comes with ethical dilemmas akin to those faced by parents. As we utilize LLMs in various domains, we must grapple with questions related to transparency, accountability, and the potential consequences of their actions.
Understanding the Motivations
To truly parent LLMs, we must delve into the motivations guiding their behavior:
Data as Parental Influence: The data fed into LLMs act as their primary influence (i.e., their upbringing). Like parents guiding their children’s moral compass, we must be cautious about the quality and biases within the data we expose LLMs to.
Feedback Loop: LLMs are trained to optimize certain objectives, such as generating coherent text or maximizing user engagement. These goals and pursuit of objectives can shape their responses and actions based on the feedback and training they receive, much like a child seeking approval from their parents or peers.
Interpretability Challenges: Understanding why LLMs produce a particular response can be challenging, given their complex neural networks. We need to develop tools and techniques that shed light on their decision-making processes, just as parents strive to understand their children’s thoughts and feelings.
The Imperative of Ethical AI Parenting: Parenting AI systems calls for an ethical and responsible approach:
We must actively work to mitigate biases in LLMs. This involves carefully curating training data, implementing fairness audits, and fine-tuning models to align with ethical standards. Developers and organizations must be transparent about how AI systems are trained, the objectives they optimize, and the mechanisms in place to ensure responsible behavior. This transparency is akin to parents setting clear expectations for their children.
Conclusion
As LLMs continue to expand their presence in our lives, our role as “parents” of these digital beings is increasingly vital. We have the power to shape their understanding of the world, infuse them with ethical principles, and guide them toward responsible actions. Much like parenting a child, understanding the “why” behind LLMs’ behavior is an ongoing journey, fraught with challenges and ethical considerations. It is a journey that requires our unwavering commitment to nurturing AI systems that positively contribute to society while mitigating potential harm. In this quest, we aim to navigate the path of AI parenting, striving to raise intelligent solutions that align with our values and aspirations.