Recently London’s Barbican hosted a fabulous exhibition by Trevor Paglen relating to artificial intelligence which gave a fascinating insight into cognitive bias.
On display were 30,000 photographs, selected from the 14 million images of the ImageNet data set, giving a visible insight into how artificial intelligence learns, with the exhibit being called “From Apple to Anomaly”. For the artificial intelligence engine worked on recognising images and starting, as we might with a small child, with “apple” when teaching the alphabet.
The result is a mosaic of images that close up rambles through connections that can be anywhere from fairly obvious through boldly humourous to feeling totally baffling and/or convoluted. Images of apples led to images of orchards but also other, reasonably distinct fruit (Banana?) and images containing a similar blush to that of a ripe Gala (Crisp and very sweet!)

What struck me most was how quickly the AI engine had turned its attention to images of people. There was initially a clear logical step from “Orchard” to “Farmer”, and the pictures of people picking apples posed no real surprise. Although the fact that people so quickly dominated the mammoth height of the Barbican’s immense “Curve” wall from the image of an apple, was surprising.
The initial band of images with people quickly fades back into images of objects but only briefly, and quite soon people become the prominent feature of all the images. And at this point biases become very apparent: manual labourers were from particular racial backgrounds; lawyers were strongly linked with traitors.
What is worse about this bias is how one incident is then treated as fact, as a sound basis for the next image selection, and the bias can not only be seen to be echoed into subsequent images but also amplified.
So it’s not just lawyers who get a bad rap but celebrities are linked to traitors, money grabbers and bottom feeders. And, possibly depending on your own personal bias, you might question the link between some celebrities and bottom-feeders and money-grabbers, whilst others may earn barely a cursory shrug as the words “fair enough” flit across the front of your mind.
Ending on an “Anomaly” is fitting given the starting point and the complex journey the images selected by the AI take you on. You would be hard-pressed to work out the start point by looking at the end. More disconcerting is the apparent ease with which the AI has travelled the various paths from “Apple”, some we can agree upon and others our own real World experience tells us to challenge – a judgement AI can’t make easily.
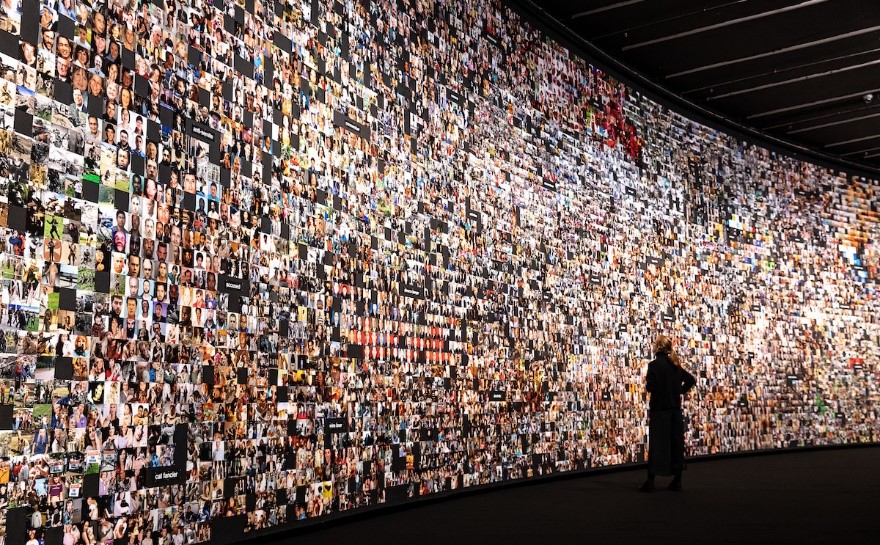
Personally, this boils down to general intelligence over specific intelligence, artificial or otherwise. Some people viewing the pictures may see connections others fail to link, and vice versa. But generally, artificial intelligence does progress from picture to picture with an apparent logic to its decision making. Where it struggles in understanding is in being able to process a nuanced view. Not all celebrities are good, not all lawyers are bad. Theoretically, at least.
The same bias happens when learning AI using the Titanic data set from Kaggle. The premise is simple – create an AI which learns how to assess is a passenger survived based on the information available, such as age, gender, and port of embarkation. The Titanic’s fateful story from 1912 is well known. However if you apply the same AI to the dataset of passengers from the Carpathia in 1918 and the results are very different. People behaved very differently as a result of the Titanic disaster, which greatly influenced who survived by comparison.
And there are plenty of other instances of artificial intelligence being swayed by bias. Early examples were biased by the influences of their creators, which was why speech recognition tended to cope better with male voices. My own attempts at the Titanic challenge have, so far, an overwhelming bias towards sending passengers to Davy Jones’s Locker.
But when focused on a single task Artificial Intelligence may, in fact, come up with surprising but right answers that people will take considerably longer to arrive to. One such example of this is Sogeti’s Cognitive QA AI engine, which can produce both conventional test reporting dashboard but also apply itself to historical test data, in doing so identifying duplicate test cases or creating in minutes a risk-based test plan tailored to the time available.