According to this McKinsey article, 70% of the organizations might adopt at least one form of Artificial Intelligence-based technology by 2030. By the end of the same decade, PWC predicts that AI could contribute up to $15.7 trillion to the global economy, which is more than the current GDP of India and China put together. Perhaps no other technology has been rapidly absorbed as AI but there are challenges at different levels as we adopt AI enabled applications and devices in our daily lives.
Inability to explain outputs
AI models work on the principle of correlation rather than causation. Due to their black box nature and complexity, AI makes it difficult to explain what factors led to a problem. Things become even more complex in the case of Deep Neutral networks or Deep Learning which could have several hidden layers and hundreds of neurons to calculate predictions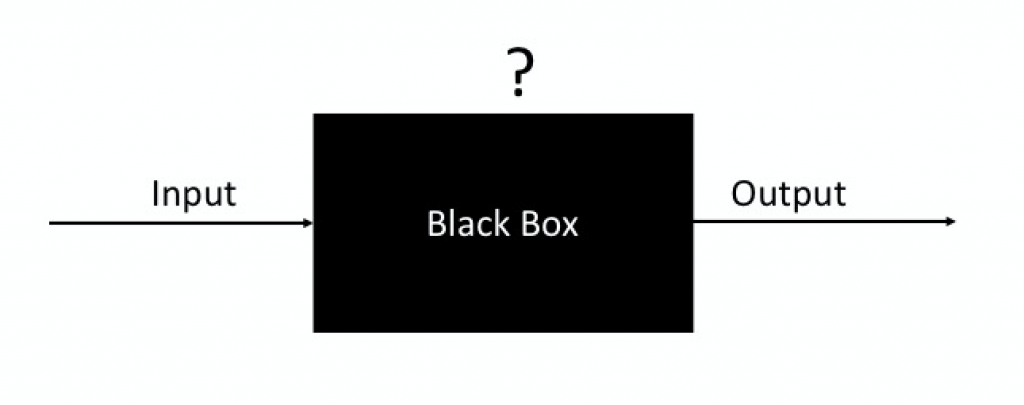