BIG DATA, ROBOTS, APOCALYPSE AND PROFIT IMPROVEMENT
July 1, 2015
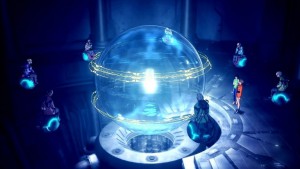
For years, I have been fascinated by the apocalyptical stories about computers and robots… and how they will steal jobs, replace ‘us’, improve themselves, rule the world, then eliminate “us,” etc. etc.!
Of course there has been few ‘stray incidents’, with some HFT bots missing the point and losing billions, a military robot going berserk and shooting bullets at friendly soldiers, a popular social network announcing a teen pregnancy, showing lack of diplomacy.
However, most of these so-called ‘fears’ have more to do with us than the technology itself. About us ‘engineers’, as we are not exactly law specialists but think that we can establish laws for such machines, instead of working with and understanding how law specialists function; about us ‘humans’ with existential doubts about our own relevance; and also, about us ‘citizens’, lost in the global / micro / macro economy.more–>
But often people seem to forget that machines are mostly an extension of our power and responsibility; they are conceived to act in a certain way and are/should be considered as such, i.e. as an extension of a human organization (sometimes a very small operation with a huge workforce). In each of the above cases, some of ‘us’ decided to take the risk of letting one of them do the wrong thing (and in most cases, one of ‘us’ was far from being equipped to handle such power and such complexity).
Now, are we all really fit to run corporations? Perhaps not all of us, perhaps not organizations of any size or complexity… Complexity is the key aspect of the matter. With growing human organizations, the growing systems of systems (or interacting machines and populations) present some unexpected behaviors. But are they really unexpected? If you let a system (composed of other smaller systems, each with unbound strategies) behave freely, you would witness a strange behavior emerge (for which you will be responsible), and some say it is not that “strange” to witness (it often is mathematically chaotic). Playing with nonlinear systems without proving, bounding and control looping anything will, unequivocally, lead to “strange behaviors” and some of them will be far from small oscillations. Doing it, ignoring these “strange behaviors” and not assuming responsibility is just silly or very reckless.
So, being responsible and making informed decisions leads, by itself, to the ability to build (i.e. manage the construction of) such complex systems. Managing mostly isolated operation support systems is one thing (where the consequences are mostly harmless), but managing interconnected control systems that embody policies, is something totally different.
Thankfully, the tools that we are building, especially in the ‘Big Data’ space seem very useful, when correctly used to work on this subject. Modeling, simulating, measuring and comparing behavior of complex systems, could help in applying correct bounds (controlled limits) to guarantee the stability in control systems.
Of course, to use them correctly, one has to differentiate action from control, cause from effect, figures from models, and not play ‘God’ too fast with the new tool at hand.
However, securing profit improvement with innovation instead of blindly moving in the mist, can make a huge difference. Certainly, this is something that will impact our business, how some services are agreed upon, and how we collaborate on a product or service that is more manageable.
We have concrete examples at hand, to stay in the (mostly) machine space:
- Modeling performance key points in a system is a simple example, which shows that applying regulation should be the norm for unpredictable load facing systems.
- Developing a model influenced by the system is a good case where the model reflects the policy, not the witnessed behavior of the system (common mistake in advertising with self-fulfilling prophecies).
- Wrongly estimating the improbable and being blind to what is required not to do also are some instances that can lead to risky (and very expensive) consequences; so, a better understanding of those aspects should be key considerations in control system design.
- Overestimating the predicting capability by mixing model, data, control and action is an example of what we shouldn’t do; in fact, it’s so common that it should make leaders raise eyebrows systematically.