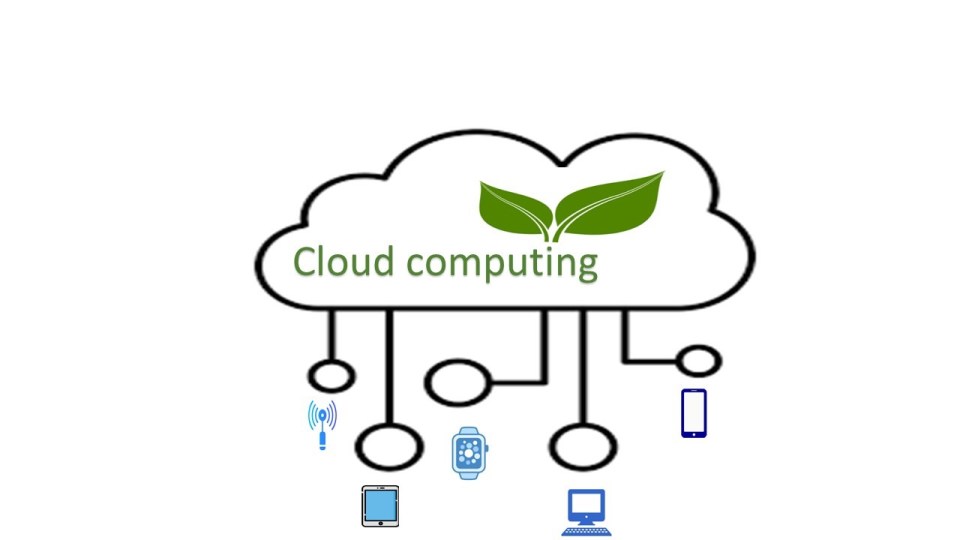
In recent years, cloud computing has revolutionized the way IT resources (e.g., storage, physical servers or virtual servers, networking capabilities, AI-powered analytic tools, etc.) are managed by individuals or businesses. In fact, cloud computing enables the on-demand availability of IT resources in the form of pay-as-you go services over the Internet. Moving to cloud offers a number of advantages including cost saving, security, scalability, flexibility and so forth. However, a study has shown that cloud computing is responsible for over 50% of all IT-related carbon emissions. To tackle this issue, it is necessary to design efficient strategies to make cloud computing greener.
Reducing energy consumption is the main idea behind solutions designed to minimize the carbon footprint of cloud computing. This can be achieved by performing strategies such as Dynamic Voltage Frequency Scaling (DVFS), data compression, resource allocation, consolidation, machine learning, server virtualization, auto-scaling, green cloud provider, network optimization, and auto-scaling. These strategies can be implemented independently or together to reduce carbon footprint of cloud computing.
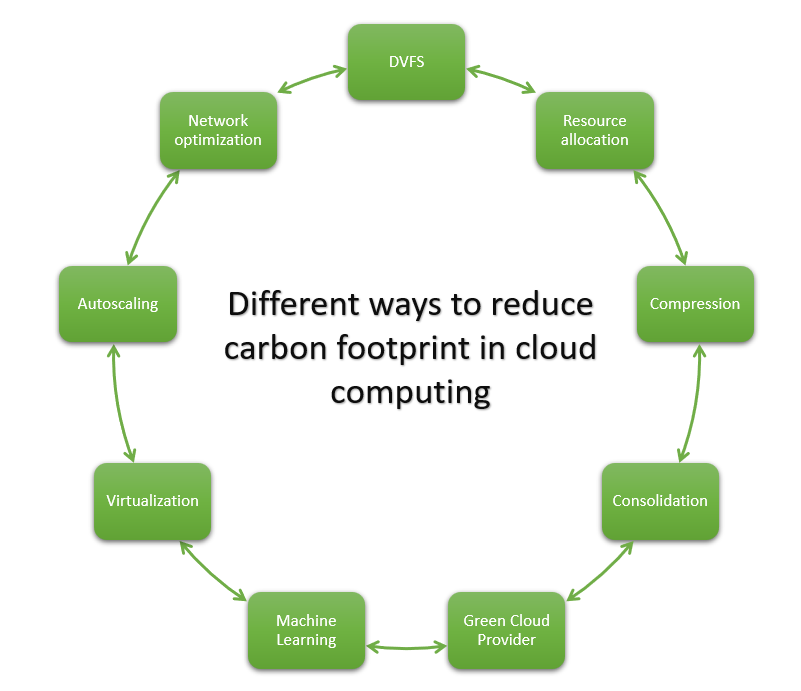
In what follows, we take a closer look at these strategies to show how they can help reduce the carbon footprint of cloud computing.
1) Dynamic Voltage Frequency Scaling
DVFS is a technique that allows the processor to modify its operating voltage and frequency to reduce power consumption. This reduction is generally achieved by adapting the processor’s voltage and frequency to the requirements of a given task. For that, frequency and voltage are set in proportion of the task’s resource requirements. In cloud computing, DVFS technique can therefore be exploited to match the resource requirements of virtual machines to the processor’s frequency and voltage. An example of a successful reduction of power consumption in cloud computing can be found in 1.
2) Data compression
Due to the significant amount of energy required to save the data in cloud computing, the data compression technique has been widely explored. This technique helps reduce the size of data to be stored or transferred, thereby reducing power consumption and carbon emissions. In practice, data compression can be achieved in two ways: a) by removing certain information to achieve higher compression ratios; b) by retaining exactly the original data. The choice of data compression strategy depends on data quality and requirements.
3) Resource allocation
Resource allocation in cloud computing refers to the task of allocating resources to cloud services in order to guarantee service level agreements. In general, resource allocation can be reactive or proactive, depending on resource utilization. Lower resource utilization means lower energy consumption. This idea is behind the development of several resource allocation approaches aimed at reducing energy consumption in cloud computing. Since the workload in cloud computing is heterogeneous and dynamic, most of the recent approaches for resources allocation in cloud computing have been developed on the basis of predictive machine learning schemes.
4) Cloud consolidation
Cloud consolidation in cloud computing is the process of merging servers or storage into one. This can be achieved through virtualization, which enables multiple virtual servers to run on a single server. The consolidation in cloud helps reduce the number of servers required, which in turn reduces power consumption and carbon emissions.
5) Machine learning
Machine learning techniques can be used to reduce the power consumption and carbon emissions in cloud computing thanks to their ability to predict future events or outcomes based on historical data. These predictive techniques can improve the reduction of the power consumption when combined with techniques such as resource allocation, job scheduling, and virtual machine consolidation.
6) Server virtualization
Virtualization refers to a technology that helps to create virtual representation of servers, storage, networks. Compared to traditional approach where a large number of physical servers is needed, virtualization helps to significantly reduce the number of physical servers. With few physical servers running, cloud service provider can reduce their power consumption and thus reduce their carbon emissions.
7) Auto-scaling
Automatic scaling in cloud computing is a feature that enables dynamic adjustment of the number of servers according to system demand. This function ensures that only the resources required by applications are used to maintain their constant availability, meet performance targeted, and minimize cloud computing costs. By minimizing the number of servers, autoscaling reduces power consumption and carbon emissions.
8) Green cloud provider
Another way to reduce power consumption and carbon emission is to choose a green cloud provider. Such a cloud provider offers up-to-date technologies and techniques that enable the minimization of carbon emissions. This includes the use efficient of cooling systems, the integration of renewable energy sources, and advanced power management techniques.
9) Network Optimization
Network optimization is the process of improving network performance using techniques such as protocol optimization, bandwidth management, compression, and content delivery networks. This process generally takes place following the identification of a problem, through prior monitoring of metrics such as power consumption, latency and bandwidth utilization. Optimizing network performance is very important, as it reduces costs and the amount of data transferred over the network, which in turn means minimum power consumption.