Overview
Generative AI affords enterprises new capabilities to enhance value creation along the customer journey across sectors. Multi-modal content generation enables exciting ways to interact and engage with customers in text, image, sound and even video formats. Real-time marketing supported by hyper-personalization and generative AI can boost an enterprise’s reach and conversion in targeted customer segments including the long-tail. The customer journey experience can go beyond conversational chatbots when powered by intelligent agent networks that act on behalf of the customer. For example, instead of expecting the customer to make a purchase decision on the spot, the customer can have a conversation with an intelligent assistant that then dispatches agents to do research to make the best buying decision or the best response to a customer support inquiry. The optimizations that can be achieved across the customer journey when applying generative AI are significant compared to existing methods.
The Customer Journey

Taking a look at the components of the canonical customer journey allows us to determine what types of business value can be created with generative AI technology. The above journey diagram illustrates the main phases in this journey that include:
- Pre-Purchase – the phase that generates awareness, interest and demand for products, services and experiences.
- Purchase – the act of following thru on the demand to obtain the best decision for acquisition of the product, service or experience, including financial terms.
- Post-Purchase – the phase where the customer uses the product, service or experience and the business provides support with any issues or inquires. The post-purchase phase also presents opportunities to continue developing the relationship with the customer with value-added propositions.
Pre-Purchase Phase
In this part 1 of the series, we’ll examine the Pre-Purchase phase to hone in on how generative AI can be used for advanced marketing capabilities to boost awareness, interest and demand. The key is to focus on business outcomes in an integrated fashion across the customer journey so that business value can be accumulated. In the journey model, this phase is comprised of the following:
- Marketing – We are most likely familiar with the function of marketing but how may we look at this set of processes in a new light given the possibilities of generative AI technology. Marketing is about generating interest and demand to incite the prospect to begin making the purchasing decision. How may we introduce the product, service and experience to someone who has never heard of the offerings? How may we make it easier to make gain traction in market segments previously cost prohibitive or not feasible in terms of time? What possibilities exist for leveraging generative AI in going after the long tail demand to increase profitability?
- Consideration – After the shopper transitions from being introduced to becoming interested, the shopper will need mechanisms to consider evaluating the value proposition before initiating the purchase. How may we enhance ways customers can conduct research and comparisons using generative AI capabilities? How may we apply generative AI to boost product-market fit so that customer needs are clearly aligned and understood? How may we use generative AI to create differentiation along key qualities customers consider such as price, brand reputation and features?

Generative AI for Marketing
For marketing one of the best ways to accelerate engagement is to make it easier for the market to search, discover and learn about products and services. One of the key advantages of generative AI is its ability to synthesize data from diverse data sources and formats and present it in a stream that can be leveraged to increase understanding faster about a particular domain. In the architecture pattern below, the power of LLMs and advanced AI-assisted search can be combined via orchestration to produce responses to product inquiries and research via a mobile or Web-based product discovery assistant.
Product information in the form of PDFs, documents, spreadsheets and even video can be used to feed the generative AI stream so that it can be shared in a chat interface driven by prompts from the user.
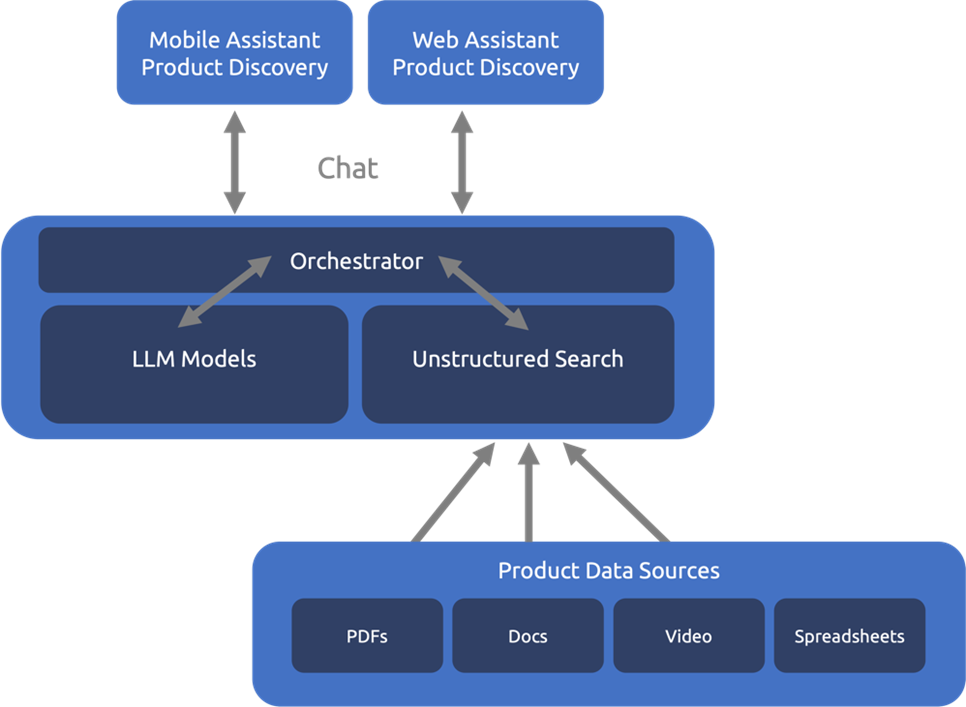
Another generative AI pattern for marketing is to consider using agents for producing marketing content for social media and customer channels. This is another powerful use case for accelerating engagement and moving potential customers along in the customer journey. Intelligent agents can leverage marketing data such as sentiment analysis to drive the creation of targeted and even personalized marketing blogs, ads, product spots and even short form video. The agents can consider marketing temporal data to optimize for delivering content at the best time to maximize engagement. These agents can also work to coordinate the marketing content related to promotional campaigns around specific market or customer segments as well as products and services.
Custom LLM models can be developed and trained to generate marketing content. Agents can perform marketing workflows leveraging custom prompts on these LLMs to automate the marketing content generating pipeline.
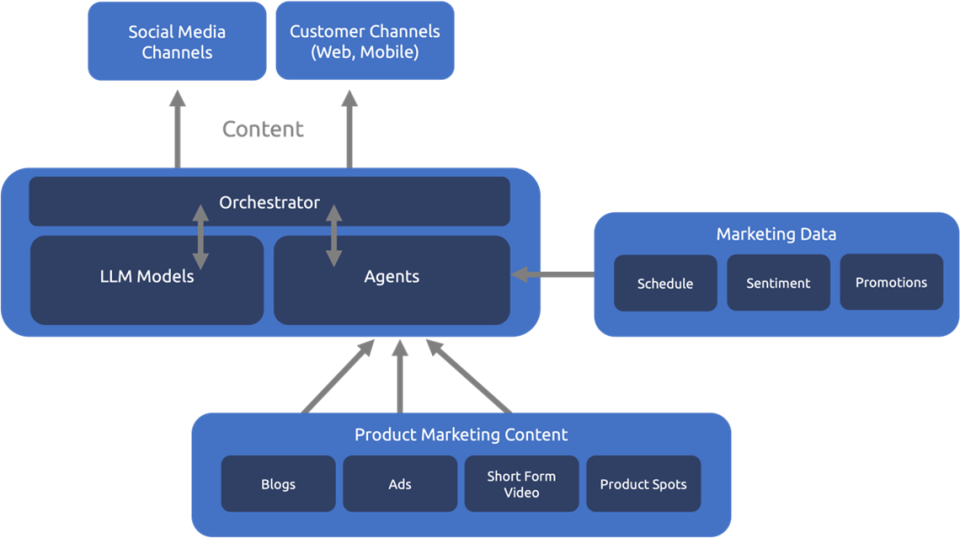
Generative AI for Product and Service Evaluation
Moving on to the consideration step, generative AI can accelerate the evaluation exercise for the prospect by making it easier to compare options and assess need fit. Using a combination of methods described for the marketing step, prompt flows and intelligent agents can be leveraged to deliver capabilities that increase effectiveness toward making the best buying decision. Often potential customers can be overwhelmed by the sheer amount of information to consider, filter and analyze to arrive at a buying decision. This friction impedes the customer journey.
Product comparison could incorporate proprietary as well as open web sources along with customer profile and history to synthesize the optimal comparison. The comparison could be increasingly effective especially over the lifetime engagement of the customer as more is known about buying habits that could be fed into the evaluation. Again, the key here is to recognize how generative AI can be applied to create responses or content to facilitate the evaluation.
Another critical component of the buying decision is the payment method. Generative AI can be applied to help the prospect to consider the best terms to fund the purchase. With so many options to provide financing, generative AI could for example, give assistance to determine which payment method to use depending on the product and service. Over time this can lead to significant savings for customers.
Other factors such as product support could be evaluated with the help of a chatbot that can provide responses to support inquiries, for instance. Upgrades and cross-grades can also be included in the evaluation.
Agents can enable an asynchronous evaluation process. Agents working on behalf of the prospect can execute an evaluation prompt workflow to compile the evaluation assessment based on the prospects own prompts about research needed to perform the evaluation. This capability is a great catalyst since evaluation can be time-consuming and likely not done in a single sitting. Agents that can get back to a prospect to share the latest details about an evaluation can serve to keep progressing toward a paying customer in the customer journey.
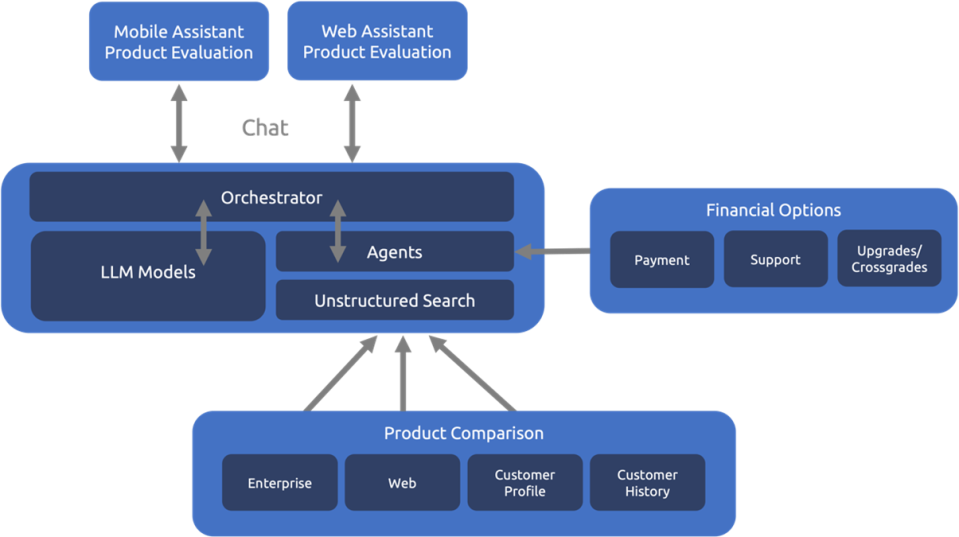
Enterprise AI Patterns
Readers may be wondering how to accelerate realizing these generative AI architecture sketches to integrate into the customer journey. One approach may be to take ad-hoc generative AI investments to develop point solutions. However, a much more optimal path is to consider generative AI as an enterprise capability which can be built upon. It is valuable to evaluate these three key factors come into play to best capitalize on business outcomes:
- Trust – How reliable is the generative AI solution and is it safe?
- Cost – What is it going to cost the business to operate the generative AI solution?
- Scale – How does the generative AI capability scale to support an accelerated portfolio of solutions across the enterprise that deliver business value?
These are the key aspects that enterprise generative AI patterns can address through its set of use case components, services and foundational operational layers. Building with consistency applying best practices is also an important enabler for maximizing generative AI investments. These services streamline provisioning and enhance reusability across a portfolio of generative AI initiatives. An integral part of these enterprise generative AI patterns are the accelerated testing and trust layers to help ensure guardrails and policies are in place to embed trust in the generative AI solution. Model and usage monitoring allow operations to determine generative AI solution performance across the customer journey. The observability is essential to tune and maximize the effectiveness of generative AI solutions. The enterprise generative AI patterns can be leveraged as a toolkit and framework to accelerate sustainable generative AI solutions that deliver business value.
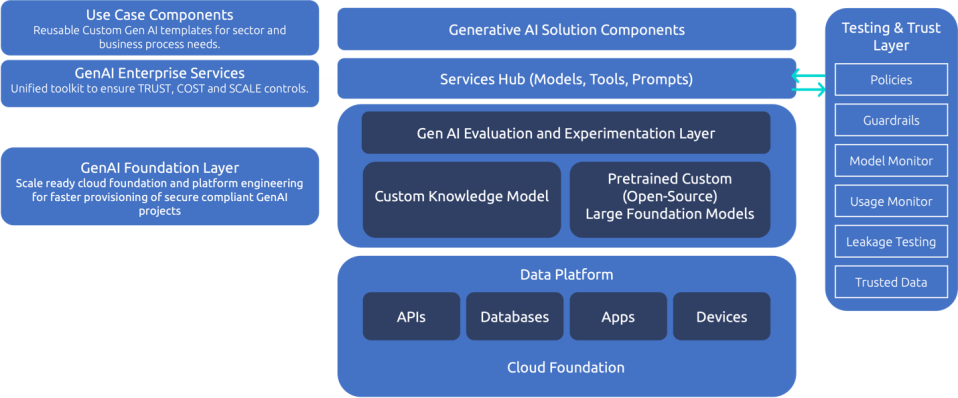
Generative AI Library Services
Enterprise generative AI patterns can be curated to provide a library of services that can be used as building blocks to implement the generative AI solutions for marketing and product discovery in the customer journey. These include security components such as prompt guardrails, prompt security classification and LLM evaluation. To enhance performance the library can include components and templates for prompt optimization, chunking, and LLM cost optimization. Agent components in the generative AI services library can be used to implement key features for the product marketing and evaluation generative AI solutions. The library includes enablement for single agent services, multi-agent systems and multi-modal agents. Core library components can be used to accelerate implementation of LLM models as services. Retrieval-augmented generation (RAG) using custom enterprise marketing data can also be integrated into the generative AI solution with these library services. RAG patterns feature significantly in the marketing and product evaluation steps described so far. RAG will play a large role as we proceed further into the customer journey.
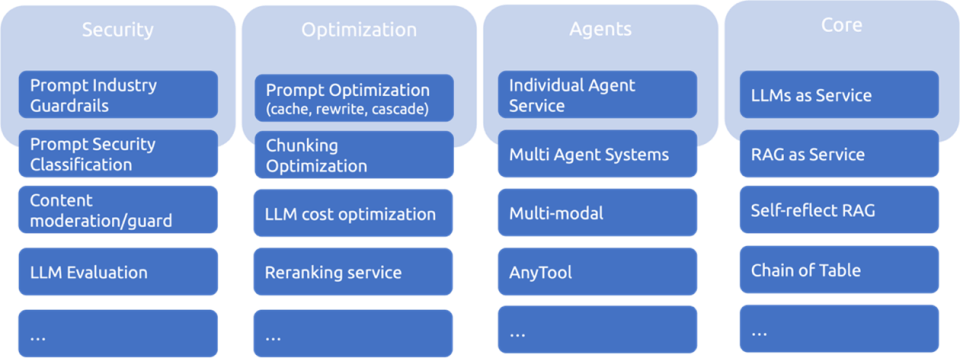
In the next part of this series, we will look at how generative AI can be applied in the Purchase phase of the customer journey. In the meantime, if you are interested in learning more about how your enterprise can set the foundation for generative AI enterprise services in the customer journey, please reach out to Sogeti to learn how we can help you achieve new business outcomes and capabilities for value creation.