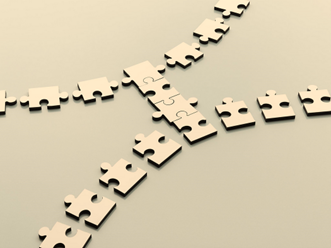
By the end of this blog, you will realize that the title itself is problematic but used for illustration purposes.
There’s an old saying that goes, “Great minds think alike, but fools seldom differ.” While thinking alike can feel validating, it shouldn’t always be the goal. Diversity of thinking brings unique perspectives and innovative solutions. In artificial intelligence (AI), however, we often expect machines to mirror human thought — aiming for AI to think like us in pursuit of “greatness.”
But as the saying suggests, if AI simply mimics human thought, we risk limiting its true potential. Human and AI “minds” don’t think alike — and that’s not a bug. It’s a feature.
This blog explores why expecting AI to achieve human-like intelligence is unrealistic and misguided. By understanding our differences, we can appreciate AI as a tool with unique strengths which can be used to augment and not replace human intelligence.
The origins of misguided expectations of AI
Spoiler alert: they are in cognitive science.
Early AI pioneers, inspired by cognitive psychology, thought replicating human cognition in machines would lead to human-like intelligence. The analogy of the brain as a computer laid the foundation for early AI research. Neural networks and symbolic reasoning were modeled after problem-solving, memory, and learning in humans.
But as AI evolved, it diverged. Cognitive science delved into understanding subjective human experiences, while AI moved toward efficiency, scalability, and specialized tasks. The hope of replicating human cognition faded as researchers realized that human intelligence is far more complex than a series of algorithms.
Human intelligence as the wrong benchmark
The ghost of cognitive science still haunts AI through our testing and benchmarks.
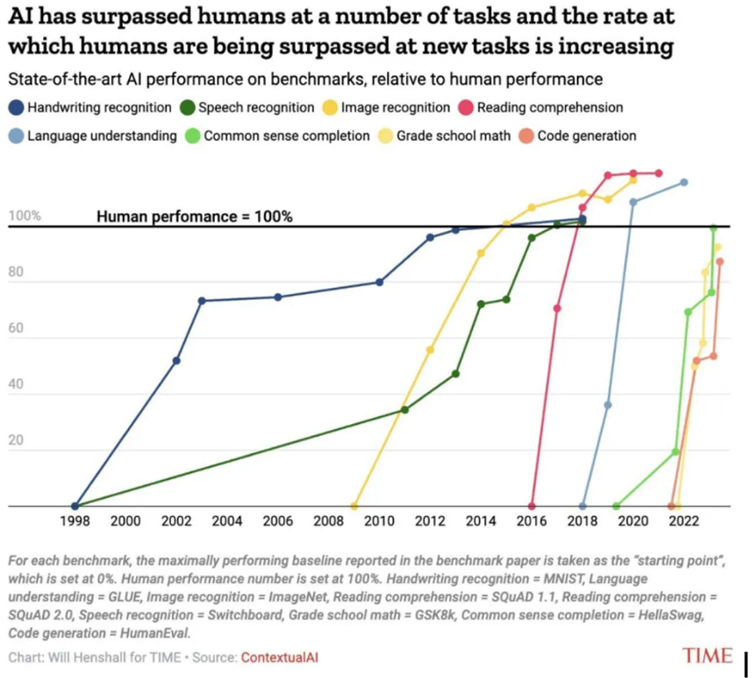
Diagram 1.0 AI’s growing capabilities as a tool for various tasks. Source.
The Turing Test set a precedent for comparing machine intelligence to human intelligence, focusing on whether a machine could convincingly mimic human behavior. But this approach fails to capture the nuance of human cognition, such as contextual understanding or creativity.
Popular LLM benchmarks test their language capabilities through tasks like question answering, coding, and commonsense reasoning. While advanced models like GPT-4o perform well on these tests, their limitations quickly emerge in out-of-context or adversarial scenarios. For instance, suggesting onions as a garnish for lemonade shows these models excel at pattern recognition but lack true comprehension.
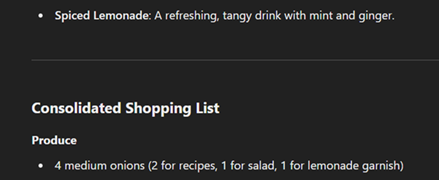
I’ve recently had GPT4o-mini tell me I should garnish a lemonade with an onion… maybe I am too closed-minded, but I decided not to take its suggestion.
Focusing on human-like benchmarks often encourages superficial improvements. Instead of testing how closely machines can mimic humans, we need tests for capabilities where AI augments human intelligence. Using sandbox environments rather than benchmarks to test would help avoid overfitting these models to a specific task.
Issues with anthropomorphizing AI
All this leads to a common pitfall nowadays — anthropomorphizing AI — projecting human traits, emotions, or consciousness onto machines. While this might seem harmless, it creates significant challenges in implementation, trust, and societal impact.

A comment on Alex’s video explaining how these models are just stochastic parrots.
In this video by Alex, we see that ChatGPT uses phrases like “I’m excited” or “I’m sorry” while answering his questions. This is easily confused with genuine emotion, while the model is just matching the question to the most likely answer based on its training data. However, when we interpret these responses as signs of consciousness, it can lead to misplaced trust or unrealistic expectations.
In fields like healthcare, anthropomorphism becomes dangerous. Algorithms are promising tools for diagnosis and treatment recommendations, but overreliance on them could be detrimental if doctors assume the AI understands patient complexities like a doctor would. Transparency in AI outputs — such as providing probabilities or reasoning steps alongside answers — can mitigate this risk by emphasizing the tool’s probabilistic nature.
Moreover, anthropomorphism has societal consequences. Media depictions of human-like robots encourage people to attribute emotions and intentions to AI. This can erode trust when the systems inevitably fail to meet these expectations. For instance, assigning AI accountability in situations like autonomous vehicle accidents could obscure the real responsibility of developers and designers, creating ethical and legal dilemmas.
To address these risks, we must treat AI as tools, not beings. Assigning non-human traits to AI in media and communication could set more realistic expectations and reduce anthropomorphic misconceptions.
Setting the Right Expectations for AI
Do I want a robot to play with my kids while I clean, or do I want it to vacuum so I can spend more time with my kids?
This question illustrates the paradigm shift we need: from replicating human intelligence to maximizing AI’s unique strengths. AI excels at augmenting human skills rather than replacing us. In radiology, for instance, AI models detect subtle disease markers in medical scans, assisting doctors without replacing their judgment. These augmentative uses show how AI can complement human intuition and creativity instead of attempting to mimic it.
However, successful implementation requires effort — not just technical, but psychological and societal. Co-creation with professionals, clear communication about AI’s limitations, and fostering trust through transparency are crucial steps to integrating AI meaningfully into our lives.
Conclusion
The gap between AI and human cognition isn’t just a matter of scale or data — it’s a fundamental difference. AI’s power lies in approaching problems from a distinct, computational perspective. Trying to replicate human cognition is both infeasible and a misdirection of resources.
Instead, we must focus on how AI can augment human intelligence. By embracing its strengths and acknowledging its limits, we can move beyond the hype of generative AI and start using these tools in valuable and transformative ways.