Today, we are facing a changing business environment. A large number of companies are considering adapting their analytical models, in order to take advantage of current technological capabilities, which are among others:
- Strong increase in cloud adoption
- Constant updates of technological tools by cloud service providers
- A decrease in the cost of storage solutions
- Increase in computational capacity through distributed processing
- The race for the adoption of Artificial Intelligence solutions
- Obtain value from the real-time analysis of the data
- Incorporation of external sources into the organization itself. Mainly these are semi or unstructured data sources, such as social networks, sensors, open data
All these factors and some others are those that are continuously “forcing” to adapt the Analytics scenario in organizations.
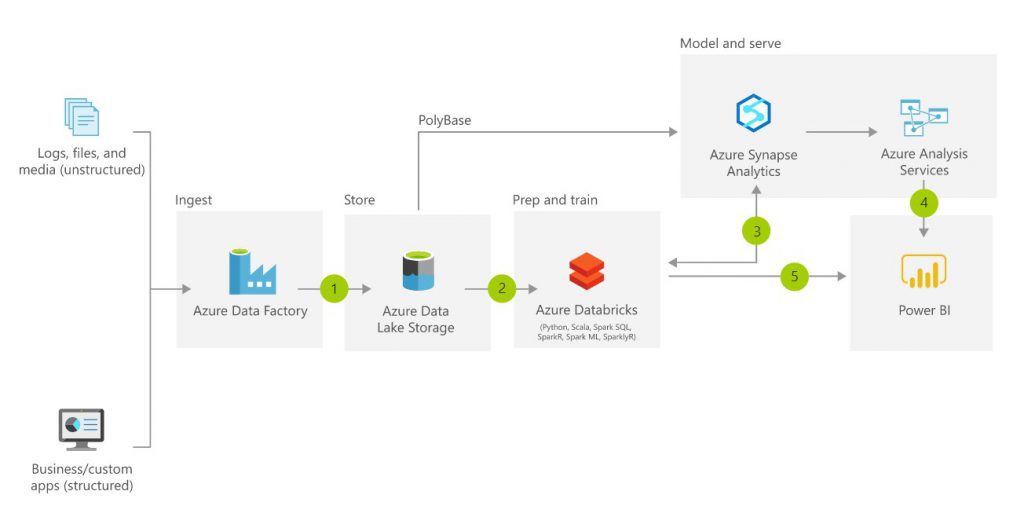
As we can see in the image above, it is a modern architecture, proposed by Microsoft, for data storage. And along with many other examples from companies like Databricks, AWS, IBM, we see that their approach to the traditional ETL changes. It goes from Extract, Transform and Load to Extract, Load and Transform, which becomes ELT.
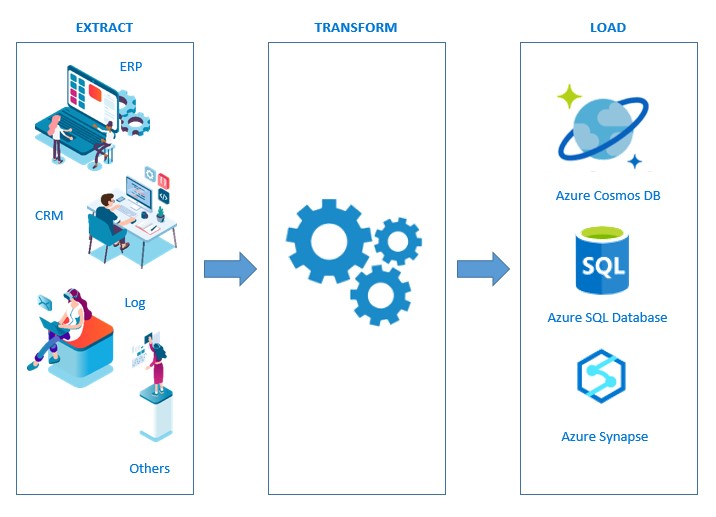
Although I have already advanced one of the key points of this evolution, I do not want to stop delving into it. As we see, this new approach to the data management process has its origin in the extraction of structural information (as in the past), but also unstructured. That together with the growth in volume, we went from MB or GB to TB, would generate real bottlenecks in the ETL tools at the time of their transformation to “leave the data pretty”.
If we add to this the reduction of the invoice for data storage in the cloud, such as Azure Data Lake Gen2 and the computational capacity of Azure Databricks, through the distributed processing of Spark. The change in strategy is clear, ELT is the winner in this case.
I don’t want to miss the opportunity to add a personal opinion towards massive data storage, indicating that it is a very good practice. Whether you have the analytical capability or not at that moment. On many occasions, when companies want to make an approach to Machine Learning, through a PoC, we find that they do not have enough historical data to make an approach with certain “guarantees”. Therefore, I recommend the storage of information. It is always better to err on the side of excess data than its lack.
So, going back to what we are dealing with. Once you have all the information in your storage account, the teams of Data Analysts, Data Scientists, Business Intelligence, will already think about the metrics, patterns, processes, projects, knowledge and benefits that can be generated from there. That will be the time to specify the data transformations, to build the best Advanced Analytics solutions for the company.
It is for all this, that the traditional ETL is giving way to ELT.
CONCLUSION
The ELT has not come to replace the ETL, both are work options that exist in the world of analytics. The choice of one or the other will depend on the model or data architecture that your company has implemented. Although, in the cases in which the intake speed is decisive, the structure of the sources is diverse and their volumes are very large, the choice of ELT is undoubtedly the appropriate one.
You can also push Databricks ML results to a storage and then consume by PBI